O
0
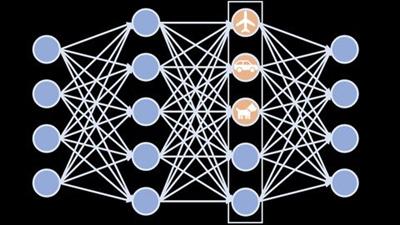
Published 12/2022
MP4 | Video: h264, 1280x720 | Audio: AAC, 44.1 KHz
Language: English | Size: 810.75 MB | Duration: 1h 49m
Neural network Simulator design in C++
MP4 | Video: h264, 1280x720 | Audio: AAC, 44.1 KHz
Language: English | Size: 810.75 MB | Duration: 1h 49m
Neural network Simulator design in C++
What you'll learn
The student will learn how to design the structure of a neural network including its neurons, bias, input layer, hidden layers, output layers and weights
The student will have a clear understanding of how the feed forward mechanism is used to shift inputs from the input layer through the output layer
The student will learn how to calculate the Root Mean Square error, output and hidden gradient, transfer function and the derivatives for a neural network
The student will also carryout back propagation on a neural network after the feed forward and use it for adjusting the weights of the neurons.
The student will learn how to generate training samples
Requirements
Theoritical Understanding of neural network, feed forward and back propagation
Basic knowledge of C++ is required
Description
This course teaches the practical design of a Neural network simulator using C++. It is recommended for all levels of C++ programmers with a theoretical knowledge of Neural network and looking forward to implement them in practice. The course interactively simulates the Neural network from the design of the class called Neuron, to the implementation of the Neuron layers in Vectors and finally the top level design consisting of the input layer, hidden layer and the output layer. Some random training samples will be generated which will be feed to the input layer through a vector and progress to the output layer through feed forward. The back propagation is also implemented which enables us to calculate the error and update the weight for a more accurate result. The training samples used in this course is for demonstration as the concept of sample generation is well explained. At the end of the course the student should be able generate real samples for testings. Some of the Core concepts we will learn in this course includes:Feed forward .Bias Neuron.Transfer function.Back propagation.Activation function.Root mean square error.Transfer function derivative.Generating training samples.Output and hidden layer Gradient.Some of the C++ concepts used includes:Assert()prototypingClass designNested VectorsReference VariablesStatic class variablesData hiding and encapsulation
Overview
Section 1: Introduction
Lecture 1 01. Introduction
Lecture 2 downloadable zip , course files
Lecture 3 02. Accessing the Number of layers and Neurons from command line
Lecture 4 03. Generating the Training samples
Lecture 5 04. Developing the Neural Network structure
Lecture 6 05. Testing our Neural Network structure
Lecture 7 06. Extracting our Training samples from text file
Lecture 8 07. Implementing the feed forward mechanism
Lecture 9 08. Extracting and displaying the results of our training
Lecture 10 09. Back propagation and calculating RMS and gradients
Lecture 11 10. Updating the weight of our Neurons
Lecture 12 11. Exercise and conclusion
All levels C++ developer
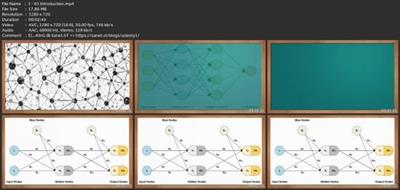
Download link
rapidgator.net:
You must reply in thread to view hidden text.
uploadgig.com:
You must reply in thread to view hidden text.
nitroflare.com:
You must reply in thread to view hidden text.
1dl.net:
You must reply in thread to view hidden text.