- Thread Starter
- #1
How LLMs Understand & Generate Human Language
Your introduction to how generative large language models work.
Overview
Generative language models, such as ChatGPT and Microsoft Bing, are becoming a daily tool for a lot of us, but these models remain black boxes to many. How does ChatGPT know which word to output next? How does it understand the meaning of the text you prompt it with? Everyone, from those who have never once interacted with a chatbot, to those who do so regularly, can benefit from a basic understanding of how these language models function. This course answers some of your fundamental questions about how generative AI works.
In this course, you learn about word embeddings: not only how they are used in these models, but also how they can be leveraged to parse large amounts of textual information utilizing concepts such as vector storage and retrieval augmented generation. It is important to understand how these models work, so you know both what they are capable of and where their limitations lie.
About the Instructor
Kate Harwood is part of the Research and Development team at the New York Times, researching the integration of state-of-the-art large language models into the Times' reporting and products. She also teaches introduction to AI courses through The Coding School. She has a MS in computer science from Columbia University. Her primary focus is on natural language processing and ethical AI.
Learn How To
Understand how human language is translated into the math that models understand
Understand how generative language models choose what words to output
Understand why some prompting strategies and tasks with LLMs work better than others
Understand what word embeddings are and how they are used to power LLMs
Understand what vector storage/retrieval augmented generation is and why it is important
Critically examine the results you get from large language models
Who Should Take This Course
Anyone who
Is interested in demystifying generative language models
Wants to be able to talk about these models with peers in an informed way
Wants to unveil some of the mystery inside LLMs' black boxes but does not have the time to dive deep into hands-on learning
Has a potential use case for ChatGPT or other text-based generative AI or embedding storage methods in their work
Download link
rapidgator.net:
ddownload.com:
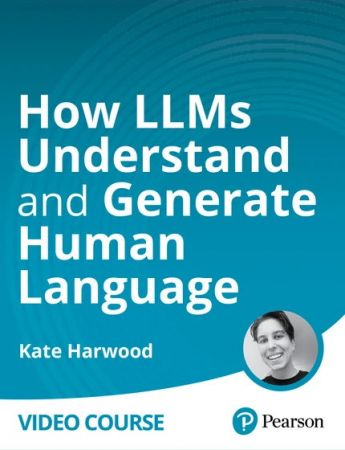
Released: 9/2024
Duration: 1h 54m | .MP4 1280x720, 30 fps(r) | AAC, 48000 Hz, 2ch | 372 MB
Genre: eLearning | Language: English
Duration: 1h 54m | .MP4 1280x720, 30 fps(r) | AAC, 48000 Hz, 2ch | 372 MB
Genre: eLearning | Language: English
Your introduction to how generative large language models work.
Overview
Generative language models, such as ChatGPT and Microsoft Bing, are becoming a daily tool for a lot of us, but these models remain black boxes to many. How does ChatGPT know which word to output next? How does it understand the meaning of the text you prompt it with? Everyone, from those who have never once interacted with a chatbot, to those who do so regularly, can benefit from a basic understanding of how these language models function. This course answers some of your fundamental questions about how generative AI works.
In this course, you learn about word embeddings: not only how they are used in these models, but also how they can be leveraged to parse large amounts of textual information utilizing concepts such as vector storage and retrieval augmented generation. It is important to understand how these models work, so you know both what they are capable of and where their limitations lie.
About the Instructor
Kate Harwood is part of the Research and Development team at the New York Times, researching the integration of state-of-the-art large language models into the Times' reporting and products. She also teaches introduction to AI courses through The Coding School. She has a MS in computer science from Columbia University. Her primary focus is on natural language processing and ethical AI.
Learn How To
Understand how human language is translated into the math that models understand
Understand how generative language models choose what words to output
Understand why some prompting strategies and tasks with LLMs work better than others
Understand what word embeddings are and how they are used to power LLMs
Understand what vector storage/retrieval augmented generation is and why it is important
Critically examine the results you get from large language models
Who Should Take This Course
Anyone who
Is interested in demystifying generative language models
Wants to be able to talk about these models with peers in an informed way
Wants to unveil some of the mystery inside LLMs' black boxes but does not have the time to dive deep into hands-on learning
Has a potential use case for ChatGPT or other text-based generative AI or embedding storage methods in their work
Screenshots
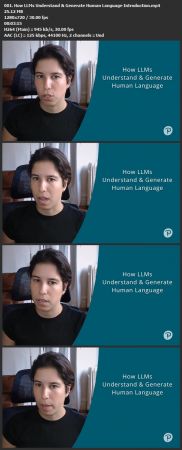
Download link
rapidgator.net:
You must reply in thread to view hidden text.
ddownload.com:
You must reply in thread to view hidden text.